PhraseCut: Language-based Image Segmentaiton in the Wild
2020년 발표된 Dataset + multimodal segmentation framework 를 제시한 논문이다.
Visual Genome의 Bounding Box annotation을 활용해, Phrase와 그에 해당되는 region을 mask annotation 했다.
Visual Genome이 어떤 dataset인지, PhraseCut은 어떤 modification을 적용했는지 알아보자~~!
** Phraes Cut의 HulaNet 모듈에 관한 설명은 해당 글에서 다루지 않습니다!
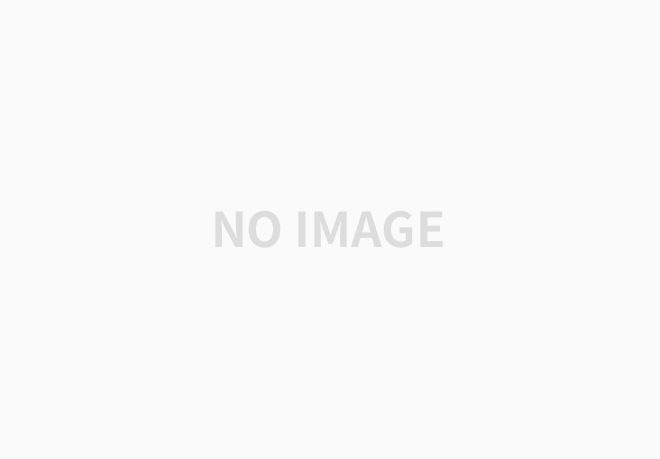
Visual Genome. PhraseCut의 baseline
PhraseCut의 base가 되는 Visual Genome dataset의 구성을 살펴보면, 한 이미지당 평균적으로 50개의 region description이 존재한다.
Description은 Object, Relationship, Attribute 3가지 내용을 포함한다.
50개의 region을 전부 visualization한 결과는 아래와 같다.
PhraseCut : Language-based Image Segmentation in the Wild
Visual Genome Dataset에 총 5개의 step을 거쳐 가공했다.
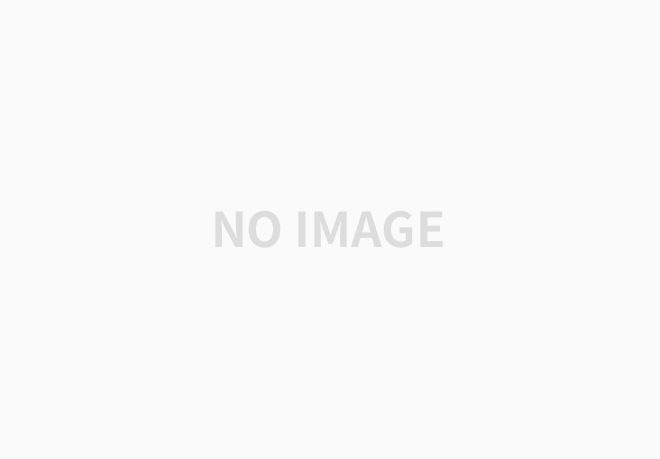
Step 1 : Box Sampling
VG(Visual Genome)의 boundung box수가 너무 많아서, 불필요한 박스들은 제거하고 평균적으로 5개의 박스를 선정했다.
- overlapping이 심한 box
- image size의 2%보다 작거나 90%보다 큰 box
- 이미 많은 sample을 보유하고 있는 category 제외
Step 2 : Phrase Generation
하나의 이미지에서 동일한 category를 가진 instance가 여러개 있을수도 있고, unique(1개)할수도 있다.
- unique한 경우 : 해당 category와 관련된 relationship/attribute를 랜덤하게 선정해 Phrase 생성
- 여러개인 경우 : 해당 category에 대한 여러 attribute 중, 특정 instance에만 적용되는 attribute이 있는지 우선적으로 탐색하고, 있다면 사용해서 Phrase 생성. 없으면 relationship으로 phrase 생성
- 모두 해당 안될 경우 : 그냥 random하게 category 선택. 이 경우에는 하나의 phrase가 여러 instance에 상응 할 수 있다.
Step 3 : Region Annotation
AWS labeling 서비스를 이용해 box -> segmentation mask로 annotation.
Step 4 : Automatic annotatior verification
Visual Genome의 bounding box와의 correspondance를 계산하는 자체 매커니즘(?)을 통해,
라벨링 결과가 별로인 worker의 결과물은 dataset에서 제외.
Step 5 : Automatic instance labeling
여러 instance들을 하나로 합치기도, 하나의 insatnce를 여러개로 나누기도, phrase에 semantic meaning에 따라 분배 및 통합한다.
ex) 사진에서 woman을 다 segment해놓고, 이후에 'three woman'이라는 phrase에 맞게 합치기.
결과는 다음과 같다. object는 물론 building같은 stuff도 정교하게 annotation이 되어 있는 모습이다.
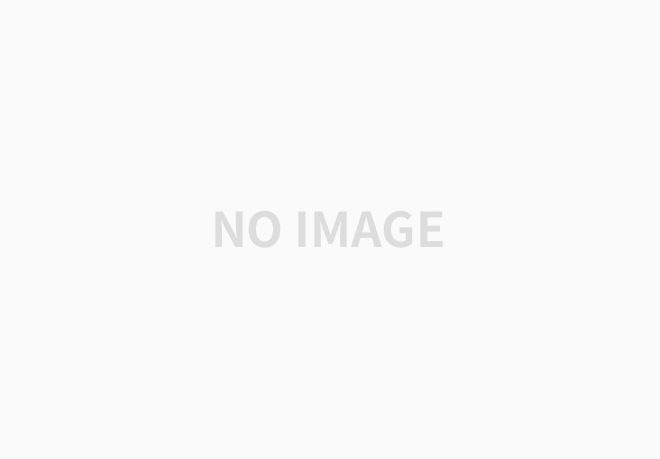